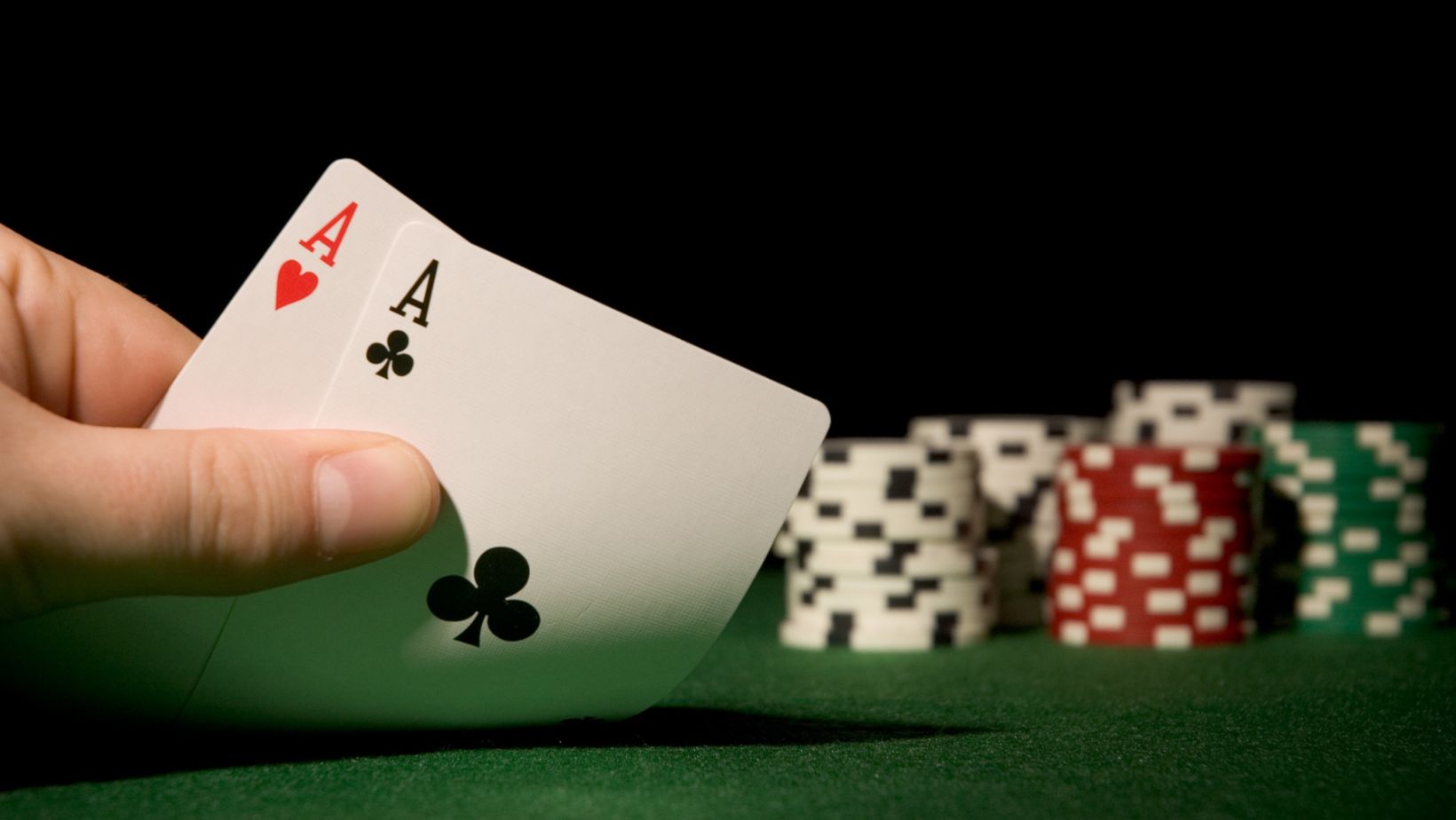
The development of online poker platforms has evolved with the integration of big data analytics. The influx of users has provided operators with a larger pool of data to analyze. It’s then used to enhance performance, study player behavior, and ultimately refine the poker experience.
Leveraging Behavioral Trends for Personalized Game Enhancements
Online poker platforms have benefited from the large volume of behavioral data generated through player actions. Poker sites can segment players into various behavioral categories by monitoring common tendencies such as betting patterns, response times, and hand frequencies. Such granularity allows the platforms to offer personalized features catered specifically to individual playing styles. In competitive environments, platforms could offer tailored advice through software that highlights a player’s weaknesses as compared to their peer group. In online poker games, a player known for cautious, defensive play might receive personalized recommendations to exploit overly aggressive opponents. Similarly, players with consistent endgame weaknesses may be introduced to training modules or insights into hand tendencies that typically lead to unfavorable outcomes. The goal is to improve a player’s strategic approach and create a more dynamic user interface based on refined predictive models from continual behavioral data analysis across millions of hands.
Data Collection and Analysis: A Foundational Approach
Poker websites collect a wealth of in-game data, including hand histories, betting actions, and chip stacks. These are essential parts in forming a robust database for analysis. The hand histories provide a comprehensive breakdown of gameplay. Meanwhile, tracking chip counts throughout a session gives insights into aggressiveness, defensive plays, and overall momentum shifts that could forecast a player’s tendencies in future hands. The methods for collecting data vary. Some sites rely on packet sniffing to capture data transmitted over networks. Others provide hand history files directly to players.
Screen scraping offers another avenue for data acquisition and gives programs access to the on-screen elements of poker games. While functional, each method brings drawbacks. For example, packet sniffing creates potential privacy concerns since the information is captured without explicit consent. Similarly, screen scraping often falters when websites update their visual elements and render scraping solutions temporarily unusable. Once the data is gathered, big data analytics, notably machine learning algorithms, are deployed to analyze millions of hands. This allows poker platforms to identify broad strategies and trends. These analyses form the backbone of the personalization strategies poker sites are increasingly embracing.
Real-Time Analytics and In-Game Decision-Making
Real-time analytics ensure players and operators can respond to ongoing game environments more easily. The availability of up-to-the-second data on betting choices, hand outcomes, and player tendencies gives players actionable insights within the same hand or game session. Tools like Super HUD provide players with a dynamic overlay of important statistics. Tools refresh real-time information continuously to inform decisions. These measures include the display of pot odds in mathematical terms or an overview of the current players’ tendencies. This helps players calibrate their next actions according to the probabilities.
Decision-making enhancements also extend into predictive analytics. AI-based odds calculators, like those embedded in advanced poker software, enable quick calculations of expected values and likely outcomes of in-game decisions. Combining real-time data with historical analysis helps players gain an immediate edge and an understanding of overarching trends such as fold chances, raising tendencies, and bluffing probabilities against specific opponent profiles.
Security, Ethical Considerations, and Concerns of Fair Play
The deployment of big data analytics has largely benefited both players and operators. However, online poker has not been immune to issues around ethics and security. The sheer amount of data generated has opened new avenues for cheating and manipulation. Operators now employ big data to detect anomalies that may suggest cheating or collusion. Unusually coordinated behavior between players or repeated anomalies in competitive play can trigger security flags. Moreover, the weaponization of big data analytics through malware has raised concerns. For example, spyware like Odlanor has been used to infiltrate players’ systems. This gives malicious actors access to private hand information. Security firms and poker platforms must offer more robust encryption measures and monitoring protocols to counteract these threats. Similarly, the ethics of profiling opponents in real-time with advanced tools is under scrutiny. Software-generated data that simplifies the targeting of weaker players sparks debates over fairness in already-competitive environments.
Players are provided with enhanced offerings through poker tools to target less-experienced players. Yet, questions about fairness arise when casual players face highly optimized opponents fueled by algorithmic insights. The extent to which these tools should be available in regulated events remains an important aspect of future discussions surrounding this blend of technology and competition. Big data analytics have revolutionized online poker by personalizing the player experience and providing real-time insights. They also raise ethical and security concerns that must be managed to maintain integrity. Balancing innovation with responsible data use will ensure a fair and enjoyable environment for all players.